Open Source vs. Commercial
TWO APPROACHES IN THE WORLD OF AI MODELS
The rapid increase in the use of artificial intelligence (AI) raises crucial questions for companies: How do you choose the right AI tool from the wide range of providers and products on offer, and is it better to opt for commercial AI services or could open source solutions be more beneficial? We have analysed these questions and now present the answers.
More and more companies are discovering AI as a way to increase their productivity and to analyse and monetise their business data more effectively. While AI-based tools are now available for almost all areas of business and tasks, marketing is currently experiencing a revolution. However, the use of AI in this area is not new.
So far, AI has been used in the context of product communication, for example, to support the management, organisation and provision of the ever-increasing volume of digital content and also to automate and accelerate recurring tasks such as keywording and the processing of image materials. Today, marketing staff in product content management can also benefit from a new generation of software solutions based on generative AI. This is a form of AI that is able to generate new content based on existing data and specifications. Text generators such as GPT-4 or image generators such as DALL-E are real game changers, especially for companies and employees who have to create thousands of product and brand contents for a wide variety of channels, and make everyday work much easier.
Against the backdrop of these new possibilities in the field of AI, however, companies are also faced with new critical questions and challenges: How do you find the right AI tool among all the providers and products on the software market? And should you rely on commercial AI services without any ifs and buts, or is an open source solution perhaps more suitable? Both options have their advantages. Below, we will contrast these and answer the question of how organisations with AI at the top of their agenda can take a balanced approach to selecting their AI tools.
Open Source AI Models
The development approach of open source AI models is open, decentralised and collaborative. Typically, the models are created as part of a community and are jointly maintained and further developed.
Open source AI models are by definition freely accessible software solutions or frameworks for machine learning. They are typically the result of collective efforts from a diverse community of researchers and developers, made available on platforms like Hugging Face along with the source code and documentation. However, companies such as OpenAI and Microsoft also offer open source models in addition to their commercial and closed source variants.
Open source AI models are generally very popular, as they do not incur any acquisition costs in the form of licence fees. However, their real strengths lie in the areas of transparency, flexibility and scalability. The open source character enables specialists to research the model architecture for their own developments or to adapt the frameworks specifically to their own requirements and needs within the company. At the same time, a lively exchange takes place on the platforms so that the models hosted there are continuously improved and innovations are driven forward by the community. However, it can also happen that open source projects come to nothing due to insufficient participation in the community and models are no longer maintained.
The most popular open source AI models at present include the BLOOM language model developed by experts from Hugging Face and volunteers from the community, Llama 2, which is the result of a collaboration between Meta and Microsoft, MPT-7B from MosaicML, Falcon from the Technology Innovation Institute (TII) and Vicuna 13-B from the Large Model Systems Organisation (LMSYS).
Regardless of who is behind the individual, freely accessible AI models, however, one problem runs through the open source landscape: the models do not always have the transparency promised by the label “open source”, especially with regard to the training data sets. While this hinders the continuous research and further development of AI models within a democratic framework, it is particularly significant when the models are used for commercial purposes. With open source solutions, the responsibility for compliance with laws and AI guidelines, which can vary greatly from state to state, lies solely with those who use them. This includes, for example, compliance with data protection regulations or copyright laws that could be violated by AI training or results.
For companies, this means that when looking for the right open source AI solution, they need to conduct thorough research into the individual models and, in addition to a specialised IT team for commissioning and maintenance, set up a team of legal experts to stay informed about the currently still very dynamic AI regulations, understand the requirements relevant to them and their solution, and thus minimise the legal risks. The open source AI models are therefore also associated with significantly higher costs than might appear at first glance.
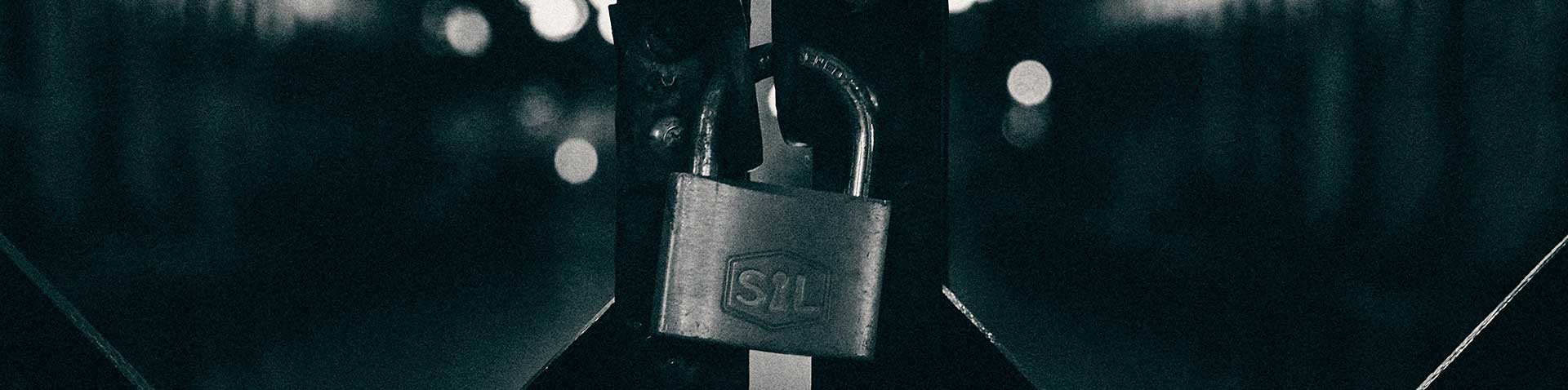
Proprietary AI Models
There is a company behind a proprietary AI model. Development is generally based on a closed source approach, which means that the source code is neither freely accessible nor can it be modified by users.
In contrast to the open source AI solutions, the commercial options are the result of targeted research by private companies. As closed source models, access must be acquired by purchasing a licence or via a subscription. As a rule, these are performance-based billing models.
The best-known GenAI tool used in marketing in general and in product and customer communication in particular is still the text robot ChatGPT from OpenAI, which was launched on the market in 2022. However, there are now a large number of solutions and models based on different GPT versions that can also process images, sound and video. In addition to OpenAI, many other well-known companies such as Microsoft, Google and Meta entered the GenAI market with the first wave – and of course the start-up scene also became active with the new possibilities.
Commercial AI services offer the major advantage that the tools are designed for broad applicability, so that they can be easily integrated into companies’ system landscapes – and users can start working immediately after logging in. They are also associated with warranty services, as well as services in the areas of change management, maintenance, further development and support. Compared to open source solutions, they can therefore be implemented and managed without the need for a specialised IT department with a large number of staff. However, legal and regulatory considerations are also important here.
For commercial AI services, the major security issues revolve around the handling of sensitive data. While the services generally offer a robust security infrastructure and tend to offer more protection than the open source variants, organisations should also put additional protocols and controls in place to avoid critical information being exposed and reused elsewhere – as well as clarifying the nuances of liability issues before purchase. After all, even with widespread providers such as Microsoft, the question regularly arises as to what extent their services are compatible with European data protection, for example. In addition, the use of AI always raises liability issues in the event of damage following incorrect results.
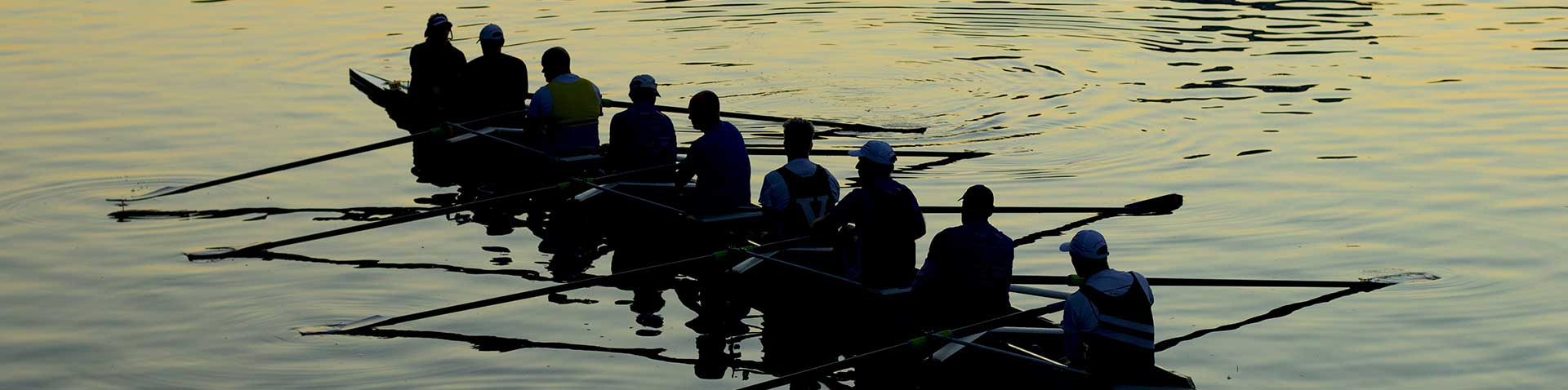
Status Quo in Product Content Management
As a software discipline, product content management continues to develop in line with technological possibilities, so the question of which development and solution approaches will win the race is a recurring one.
The topic of open source has also arrived in product content management. More and more software manufacturers are offering licences for community open source products with core functions such as MDM, PIM and DAM for a usage fee, which can be expanded to include modules, functions and services for additional licence fees. This so-called enterprise open source approach thus appears to combine the advantages of both worlds: companies can get started at low cost, adapt their product content management solution freely or depending on the open source licence, benefit from the provider’s role as patron in terms of stability and security risks and can decide flexibly on further investments, such as the integration of an AI model. However, consumers should not be blinded by these typical marketing promises. Whether the price-performance ratio exceeds that of classic proprietary solutions in the long term depends heavily on the company itself and the possibilities and needs of the organisation.
At the same time, an opposing trend in product content management is emerging on the software market: so-called out-of-the-box solutions are becoming increasingly popular. These are ready-to-use software products that are designed to appeal to a broad market base and are tailored to industry requirements. While these solutions do not provide the customisation that we see in the customised approach of proprietary solutions, or the flexibility that comes with community or enterprise open source solutions, the product type has the advantage of evolving with industry and market requirements and associated technology trends, so that GenAI functions can be used in a variety of industries and markets. PIM and DAM are already part of the basic equipment of some providers and do not need to be purchased.
Management Summary
Regardless of the advantages and disadvantages mentioned, the decision in favour of or against a commercial or open source solution is a strategic one that must be made depending on the company and its individual capabilities and specific needs and requirements.
Open source solutions offer the possibility of closely aligning the model with business requirements and scaling it further. However, this strength can only be realised if companies have the necessary human resources or are prepared to invest in their IT department and experts accordingly. In addition, the open source approach requires an extremely robust internal infrastructure for operational management and security issues. These aspects and cost items must therefore also be taken into account in the cost-benefit analysis when deciding in favour of such a model.
Commercial services, on the other hand, and especially those solutions that are geared towards business use, are integration-friendly and do not involve such entry barriers. As they are generally customised to industries and specific areas of responsibility and the purchase is accompanied by various services, they also score highly in terms of user-friendliness at all subsequent levels. However, the extent to which the acquired AI solution delivers added value and contributes to increasing productivity, for example, depends not least on its architecture and how it is used. The solution must be seamlessly integrated into the existing system landscape and established workflows so as not to create any new problems during ongoing operations – and to be able to provide comprehensive support for the processes. To avoid a patchwork-like collection of solutions, it is also advisable, especially in very complex business areas such as product communication, to use overall solution approaches that cover all relevant areas of responsibility and integrate state-of-the-art technologies such as Gen AI.
Picture credits: Getty Images on Unsplash; Mitchell Luo on Unsplash; Adam J. on Unsplash
Would you like the article as a PDF file?
HERE YOU CAN DOWNLOAD THE ARTICLE IMMEDIATELY.